New technologies have been shaping the music world since its inception. From the advent of the first recordings, through the electronic revolution, to today’s AI technology, the role of technology in the creative process has been continually increasing. Many groundbreaking events that pushed the boundaries of the music world have occurred thanks to decades of research and discoveries in the field of artificial intelligence. In this article, we will trace the evolution of AI music methods to see how this technology creates new sounds, melodies, develops entire compositions, and even imitates human singing.
- Early Stages of AI Development in Music (1950s – 1970s)
- Transition Period of AI Development in Music — Generative Modeling (1980s – 1990s)
- Current Stage of AI Development in Music (2000 – Present)
- Intelligent Composition with Iamus
- Musical Analysis with Project Magenta
- Sound Synthesis with NSynth
- Generative Modeling with Jukebox
- Further Breakthroughs in AI Music Evolution
The role of artificial intelligence in understanding and creating music has significantly increased since the 1950s. From basic algorithms to a multifaceted industry with intelligent music systems, the progressive development of AI music demonstrates the technological expansion of this methodology.
Early Stages of AI Development in Music (1950s – 1970s)
The first attempts at computer-generated music appeared in the 1950s, focusing particularly on algorithmic composition. This new kind of music, pioneered by figures such as Alan Turing with the Manchester Mark II computer, opened many possibilities for researching musical intelligence, using computational systems capable of recognizing, creating, and analyzing musical pieces.
Early experiments concentrated especially on algorithmic composition, where a computer creates music based on formalized sets of rules. In 1957, the first piece composed entirely by artificial intelligence, the Illiac Suite for string quartet, was created.
Using mathematical models and algorithms, American composers Lejaren Hiller and Leonard Isaacson created the Illiac Suite, the first piece entirely composed by a computer. They used the Monte Carlo algorithm, which generated random numbers corresponding to specific musical features such as pitch or rhythm. By using a set of restrictive rules, these random features were limited to those considered “correct” from the perspective of traditional music theory, statistical probability (Markov chains), and the composers’ imagination.
Another innovator in this field was Iannis Xenakis, a composer and engineer who used stochastic probabilities in his own work. A stochastic process is a mechanism with a random probability distribution that cannot be precisely predicted but can be analyzed statistically. As early as the 1960s, Xenakis used a computer and the FORTRAN language to program a set of probability functions that determined the overall structure and various properties of a composition (such as pitch and dynamics).
Xenakis modeled his music as if it were a scientific experiment, where each instrument was a particle subject to a stochastic, random process determining its behavior (pitch and dynamics of individual notes).
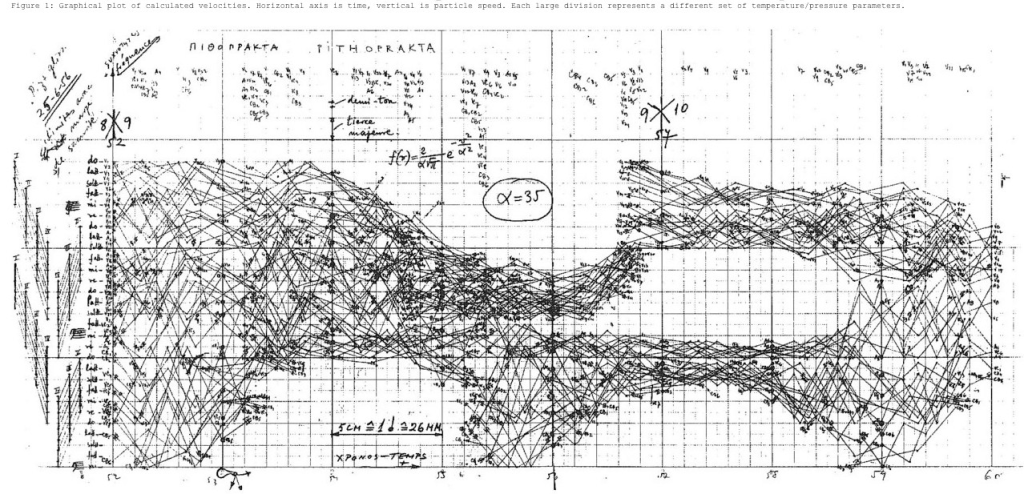
His work served as a starting point for many new methods of music creation and as an early example of using artificial intelligence as an analytical tool, not just a compositional one. Xenakis’s method of creating melodies and orchestrating for different instruments was based on sound spaces modeled by stochastic processes.
This duality of artificial intelligence as an autonomous creator and a supportive tool persists today. Among intelligent systems, we can distinguish those that specialize in generating new pieces, like the Illiac Suite, and those that use stochastic and analytical methods, as Xenakis did.
Transition Period of AI Development in Music — Generative Modeling (1980s – 1990s)
In the decades preceding the modern era of music, the focus shifted from simple, algorithmic music composition to generative modeling. Otto Laske, a prominent researcher in sonology, describes this shift as the difference between a musical robot and musical intelligence.
A musical robot operates similarly to early experiments from the 1950s and 60s—it recognizes patterns, knows musical grammar, and has general problem-solving skills but achieves its goals using relatively straightforward and clumsy methods. On the other hand, musical intelligence replaces this robotic methodology with a knowledge-based system that understands the functioning of various musical elements.
This trend towards developing AI systems that build their own understanding of the meaning of individual musical elements became the foundation for the evolution of musical intelligence to the higher level we see today.
David Cope, a composer and music professor, conducted his own experiments with musical intelligence (EMI) in the 1980s. He strongly believed that computer composition could encompass a deeper understanding of music through three fundamental methods:
- Deconstruction (analysis and breakdown into parts)
- Signature (maintaining elements defining a musical style)
- Compatibility (recombination—reassembling musical elements into new works)
His work revolved around the idea of recombination, where elements from previous works are combined and modified to create new musical pieces. Some of the greatest composers of all time also used recombination (consciously or not), transforming existing ideas/styles into new works. Cope aimed to replicate this methodology within the EMI program, using computers and their computational abilities.
Cope’s work became the basis for many of today’s AI models. Initially, music and its attributes are encoded in databases. Then, a set of recombined segments is extracted using specified identifiers and pattern-matching systems. In subsequent steps, musical segments are categorized and assembled into a logical, musical order using extended networks until a finished piece is produced. This concept of “regenerative” music construction resembles how neural networks independently compose musical pieces today.
Further achievements of this period pushed the boundaries of computational creativity. For example, Robert Rowe developed a system that could infer the meter, tempo, and length of notes from a “live” performance of a piece. In 1995, Imagination Engines trained a neural network using a set of popular melodies and applied reinforcement learning, resulting in the generation of over 10,000 new musical motifs. Reinforcement learning involves training a neural network to achieve a specific outcome by rewarding or penalizing the model based on the accuracy of its decisions.
Current Stage of AI Development in Music (2000 – Present)
At the current stage of AI development in music, the roots of generative modeling and algorithmic composition have spread dynamically into higher-level research and even the entire music industry. Thanks to the use of more experimental algorithms and deeper neural networks, the role of musical AI in the creative process has significantly increased.
Intelligent Composition with Iamus
Founded in 2010, Melomics Iamus was the first computer capable of composing classical music in its own style. Iamus is a computer cluster that uses evolutionary algorithms to compose musical motifs, showing some deviations from Cope’s generative model, which relied on existing works.
Like the process of natural selection, a randomly generated musical piece undergoes mutations (altered sound, dynamics, etc.). This evolution allows a random input fragment to develop into hundreds of compositions meeting the criteria of fully-fledged music within minutes.
Musical Analysis with Project Magenta
Magenta, a project initiated by Google Brain, uses machine learning to enhance the creative process. It has produced a series of applications demonstrating musical intelligence capabilities, such as transcribing sounds using neural networks or combining musical scores with latent space models. The depth of musical analysis conducted by Magenta can be seen in experiments with MusicVAE.
MusicVAE is a machine learning model that composes musical pieces by combining scores. It does so by using a hidden spatial model, where a multidimensional dataset is translated into a more comprehensible mathematical language. An autoencoder performs this by compressing (encoding) each element of melodies into a vector form and then converting that vector back into a melody (decoding).
After learning how to compress and decompress input data sets, the autoencoder identifies common features across the entire set. MusicVAE operates on this principle but introduces hierarchical structures that create a summary structure.
Working within this structure, MusicVAE can construct applications for creating interpolations, drum patterns, and entirely new melodic loops based on musical input data.
Sound Synthesis with NSynth
While most early research focused on the composition process, current experiments have extended to machine learning in sound synthesis. Magenta NSynth (Neural Synthesizer) uses neural networks to create sounds at the level of individual samples rather than using oscillators/wavetable synthesis like traditional synthesizers. This approach allows for greater artistic control over the final timbre (the specific character of a sound), significantly aiding the creative process.
NSynth uses a WaveNet-style autoencoder working on a dataset containing 300,000 musical samples from around 1,000 instruments. This unique dataset allows the factorization of musical sound into individual notes and other features based on dependencies from the law of total probability.
The autoencoder’s task is to model the timbre of sound—P(audio | note) in the above equation—in the latent space. NSynth employs a temporal coder with mathematical algorithms known as convolutions. After passing through 30 layers of these convolutions, both input notes are compressed into 16 dimensions corresponding to 16 sound features in the time domain. The compressed data are then upsampled and interpolated, resulting in new embeddings (mathematical representations of sound). The final step involves synthesizing a range of new sounds carrying characteristics of both input sounds by decoding these embeddings.
Generative Modeling with Jukebox
Most experiments in autonomous music composition tend to generate music symbolically, often using piano roll editors or MIDI as the language for describing sounds/sequences. OpenAI’s Jukebox takes generative modeling to a higher level by directly modeling music and human voice as raw audio. This approach allows Jukebox to create melodies, compositions, timbres, and even simple singing across various genres and styles. To handle the semantic depth of raw audio, Jukebox employs specialized encoders and neural networks.
Jukebox compresses audio into a latent space using VQ-VAE encoders. The processing chain begins with encoding the audio using convolutional neural networks (CNNs), followed by pattern recognition in the results. A CNN is an algorithm that can take an input image and represent its unique features mathematically using multi-dimensional matrices. CNNs can also be applied to audio to create a visual representation of an audio sample, such as a spectrogram.
To mitigate the loss of musical data during encoding, Jukebox uses a combination of loss functions and upsamplers to retain information. These patterns are then decoded into new audio using more CNNs.
However, Jukebox differs from other models due to the nature of VQ-VAE, which is essentially an autoencoder with a “discretization bottleneck” feature. This means it preserves varying amounts of musical information across three independent reconstruction levels (each encoding different amounts of information). This use of separate encoders instead of a hierarchical system allows the model to better handle the latent space of raw audio, as the sound can be reconstructed from any of these three layers.
A comparison of spectral reconstructions from different VQ-VAEs, with time on the x-axis and frequency on the y-axis. The three columns represent different reconstruction levels. The top layer shows the actual source audio. The second row presents the effects of Jukebox’s separate autoencoders. The third row shows results without spectral loss functions. The fourth row displays hierarchical VQ-VAE results. The fifth row shows the output of a similar Opus codec. Image by OpenAI.
Trained on a dataset containing 1.2 million songs, Jukebox represents a significant advancement in generative music modeling. Maintaining musical coherence, adherence to traditional song structures, authenticity in solo parts, and replication of human voice in generated tracks, it shows clear progress over early ideas of recombination or algorithmic composition.
Further Breakthroughs in AI Music Evolution
The continuous development and increasing presence of artificial intelligence in music have led to numerous commercial applications. For instance, the service LANDR uses deep learning algorithms for automatic audio mastering. Additionally, combinations of neural networks and reinforcement learning algorithms have been employed in creating commercial recordings, such as Taryn Southern’s album (co-produced by Amper Music) and the Hello World album (produced using Sony’s Flow Machines with various artists). As these algorithms and neural networks evolve, more AI-assisted music is expected to appear in mainstream media soon.
Since the pioneering work of researchers like Hiller/Isaacson and Cope, the sophistication of AI technology has steadily increased, and its adoption in the music industry has surged. Commercial applications now assist artists in the creative process, including film music and background scores. However, the role of the human mind remains crucial for preserving true emotionality and creative depth in music.
There is no doubt that the growing accessibility and capabilities of AI will transform the music industry. However, rather than being helpless in the face of these inevitable changes, musicians can learn to harness AI as the next step in their creative evolution, adapting their work processes accordingly.